Grasping the nuances between machine vision and computer vision can feel like a maze, right? If it’s any consolation, you’re not alone. In my own exploration, I uncovered that while machine vision shines in industrial contexts, computer vision casts its net much wider across various applications.
This article aims to simplify these intricate subjects into manageable chunks for an easier understanding. Ready to get started? Let’s peel back the layers together!
Key Takeaways
Machine vision uses special cameras and software to perform precise tasks in industrial settings, like inspecting parts or guiding robots.
Computer vision helps machines understand images like humans do. It can analyze visuals from any source for a wide range of applications, including self-driving cars and augmented reality.
While machine vision focuses on real – time processing for specific tasks, computer vision learns from historical images to recognize patterns and objects.
Table of Contents
Definition of Machine Vision
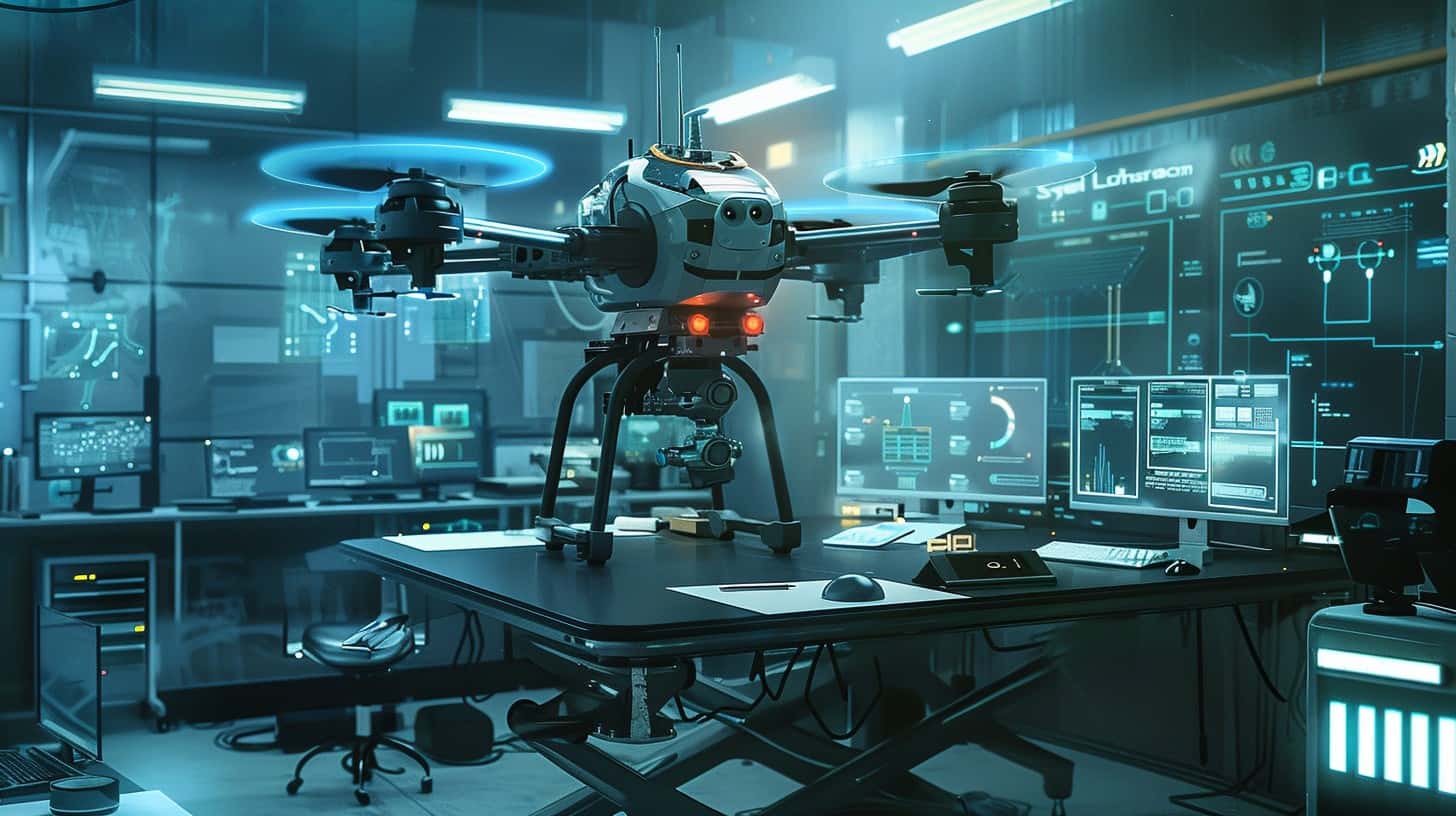
Machine vision involves technology and methods focusing on processing visual input in industrial settings. It uses specialty cameras to capture images, then processes this information for tasks like visual inspection and object detection.
Think of it as the eyes behind automating complex or repetitive tasks where precision is key.
This tech hinges on high-quality cameras capturing environmental images—processing these snapshots based on predefined aspects. It’s not just about seeing; it’s about analyzing with purpose, whether that’s identifying defects on a manufacturing line or ensuring robots place components just right.
Now, let’s dive into how machine vision actually works.
How Machine Vision Works
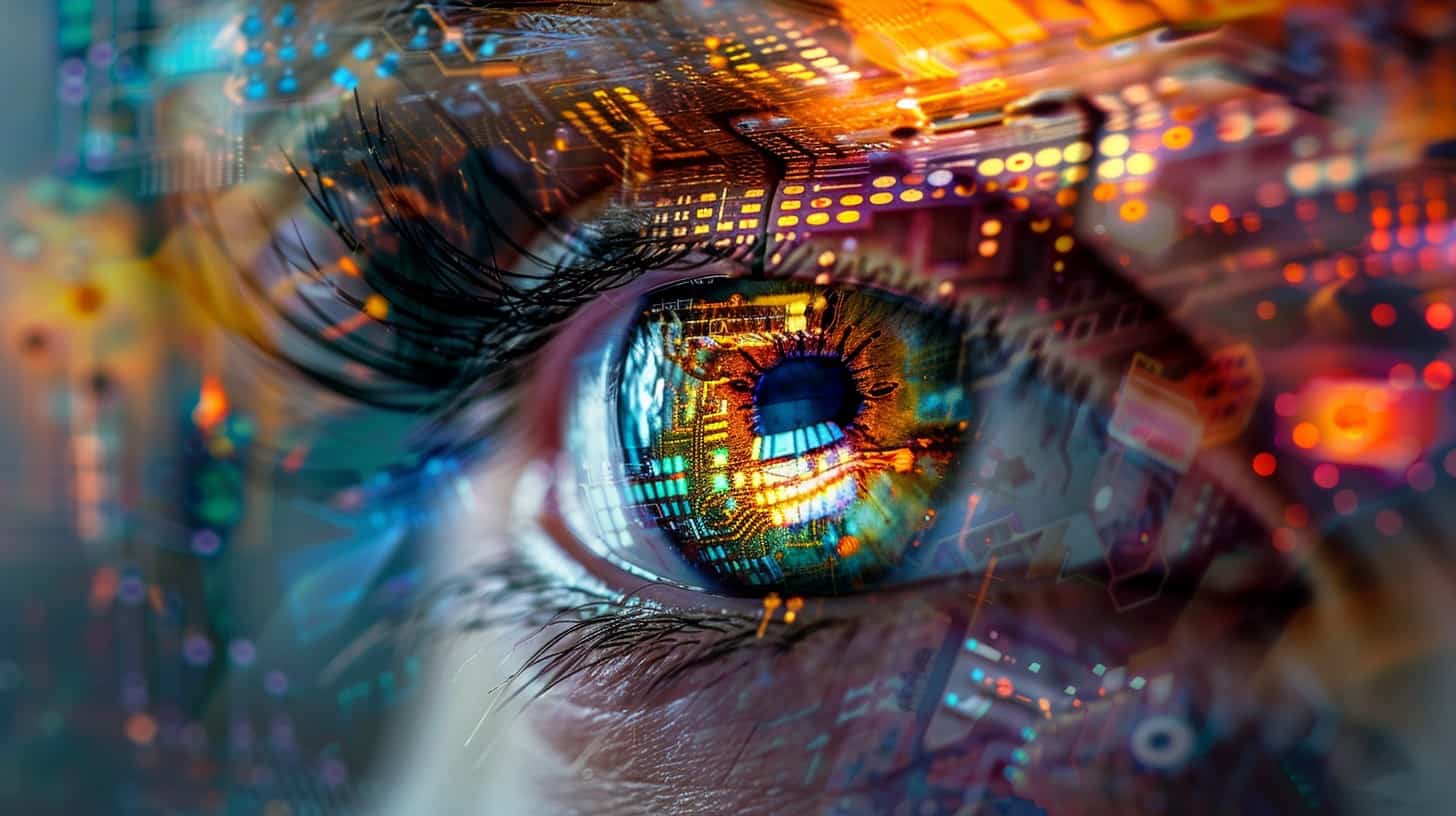
Capturing images kicks things off. Specialty cameras designed for machine vision do this job, grabbing visuals of what’s in front of them. These aren’t your average snapshots, but high-quality digital images meant for analysis.
Once captured, the real magic begins with processing these images. Think of it as feeding information into a brain wired to notice details humans might miss. This process isn’t casual browsing; it’s rigorous and precise, driven by software set up with very specific instructions.
After the initial capture, object detection or visual inspection comes into play. Here’s where strict parameters from the software guide the system to make sense of what it sees. It sounds straightforward, but imagine teaching a computer to recognize flawed products on an assembly line or sort items based on tiny distinctions—tasks that demand not only sharp eyesight but also relentless consistency and speed.
That’s machine vision at work, confined to interpreting scenes through its lenses and making automated decisions without human intervention. This integration within larger systems ensures operational efficiency—a game-changer for industries far and wide.
Applications of Machine Vision
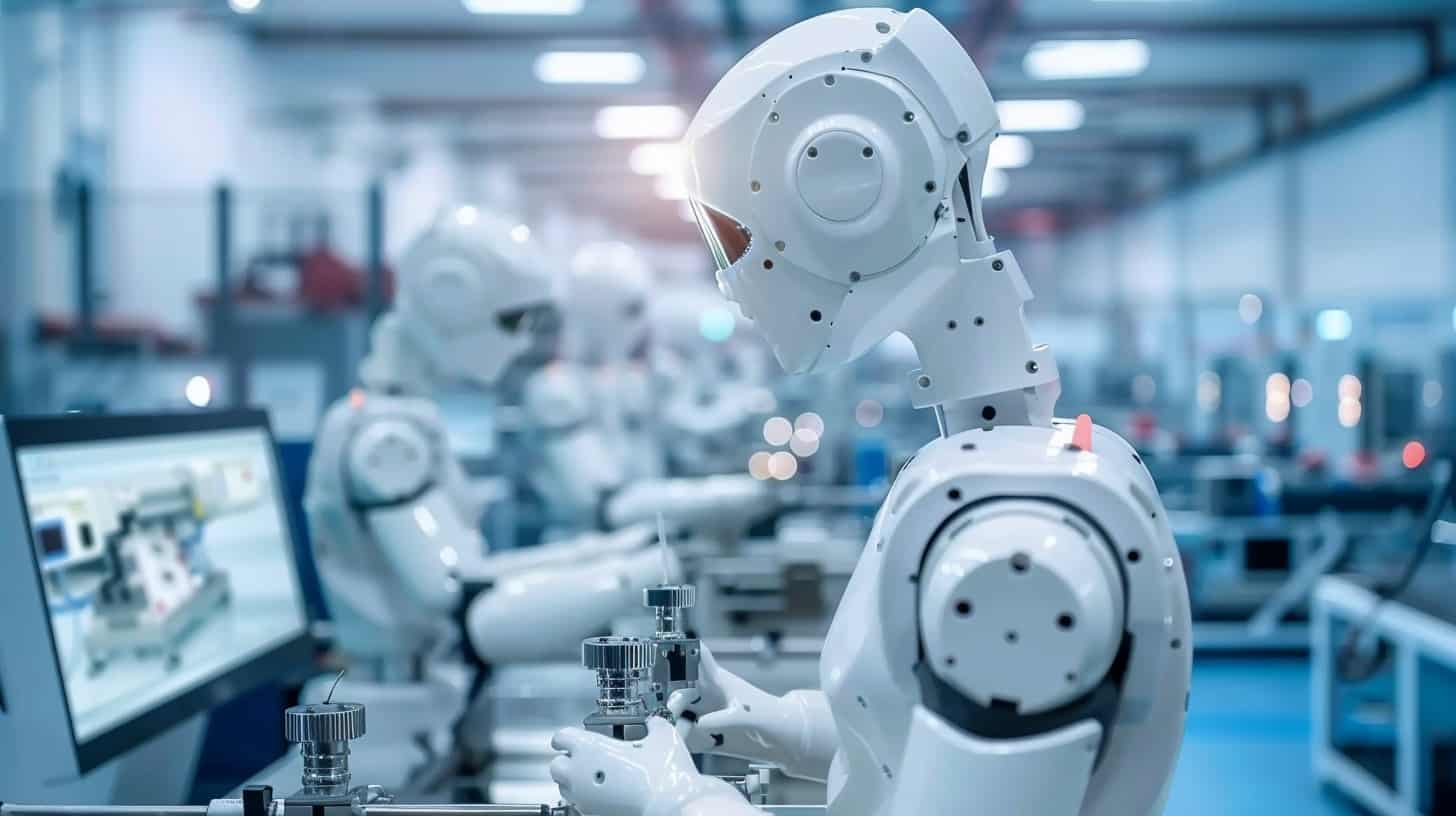
Machine vision is revolutionizing the way robots, manufacturing processes, and industrial applications function, making them more intelligent and efficient. This transformation is supported by advancements in computer vision development services, such as those provided by companies like Requestum.
By leveraging state-of-the-art technologies, Requestum helps businesses integrate machine vision solutions that enhance automation, improve quality control, and increase productivity. Dive deeper to explore the vast world of machine vision innovations and discover how computer vision development services are paving the way for smarter, more effective operational frameworks across various industries.
Robots
Robots have become essential in today’s tech-driven world, especially where precision and efficiency are critical. With machine vision, robots can see, identify, and interact with their environment more accurately than ever before.
This technology powers robots in manufacturing plants, helping them assemble parts with incredible speed and accuracy. It’s fascinating to see how these machine-equipped robots perform tasks that were once deemed impossible without human intervention.
In industries like automobile manufacturing and e-commerce warehouses, computer vision elevates the role of robots significantly. These intelligent machines rely on advanced image recognition technologies to automate production lines and manage stock operations seamlessly.
Their ability to learn from supervised learning algorithms enables them to improve over time, making processes faster and more cost-effective. Seeing a robot equipped with computer vision navigate through a warehouse or assemble a car part is a clear indicator of how far we’ve come in integrating AI into practical applications.
Manufacturing
In manufacturing, machine and computer vision systems revolutionize how things are made. OnLogic offers specialized computers perfect for these tasks. They’re a game-changer in industries like automotive manufacturing.
Here, precision is key—every part must fit perfectly. Machine vision helps by inspecting each piece, ensuring it meets high standards before assembly.
Automobile manufacturers lean heavily on this tech. With computer vision powered by machine learning, automated production lines become faster and more efficient. It’s all about minimizing errors while maximizing speed.
These systems guide robots to assemble cars with accuracy that matches—or beats—human hands.
Industrial Applications
Leaving manufacturing behind, we dive into the broader scope of industrial applications. Machine vision systems are power players here, crucial for tasks like quality assurance and object sorting.
These systems check products at lightning speed, catching defects before they leave the factory floor. This isn’t just about making things look good—it’s essential for safety and reliability across industries.
On another front, computer vision is transforming how we approach security and monitoring in these spaces. Think about it—cameras that can identify hazards or unauthorized individuals in real-time.
It’s like having an extra set of eyes that never blink, equipped with AI to make instant decisions. Both technologies are vital—keeping production lines efficient and workplaces safe without missing a beat.
Definition of Computer Vision
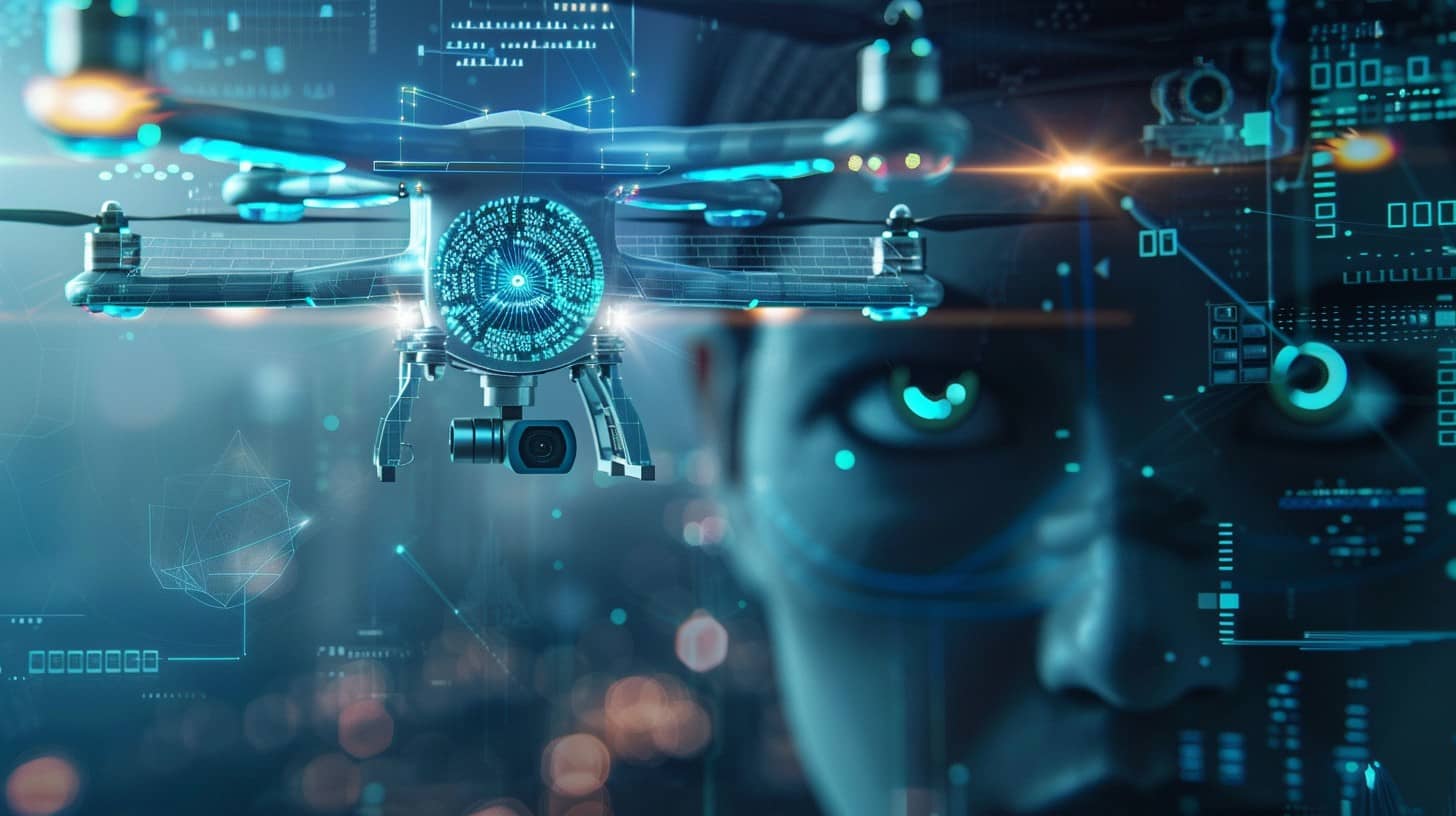
Computer vision is a part of artificial intelligence that focuses on enabling computers to interpret and understand visual information the way humans do. It’s about teaching machines to process and analyze images or videos to automate tasks or provide insights.
This technology uses digital image processing and machine learning algorithms to recognize patterns, objects, or actions in visual data.
It deals with various forms of visual inputs–from cameras, motion detectors, thermal sensors, radar, to LiDAR systems. By analyzing this data, computer vision can perform a wide range of tasks like identifying objects in real time or tracking movements within a video feed.
The goal is clear: make computers see as well as humans for applications stretching from enhancing safety in manufacturing floors through live video analysis to powering object recognition capabilities in autonomous vehicles.
How Computer Vision Works
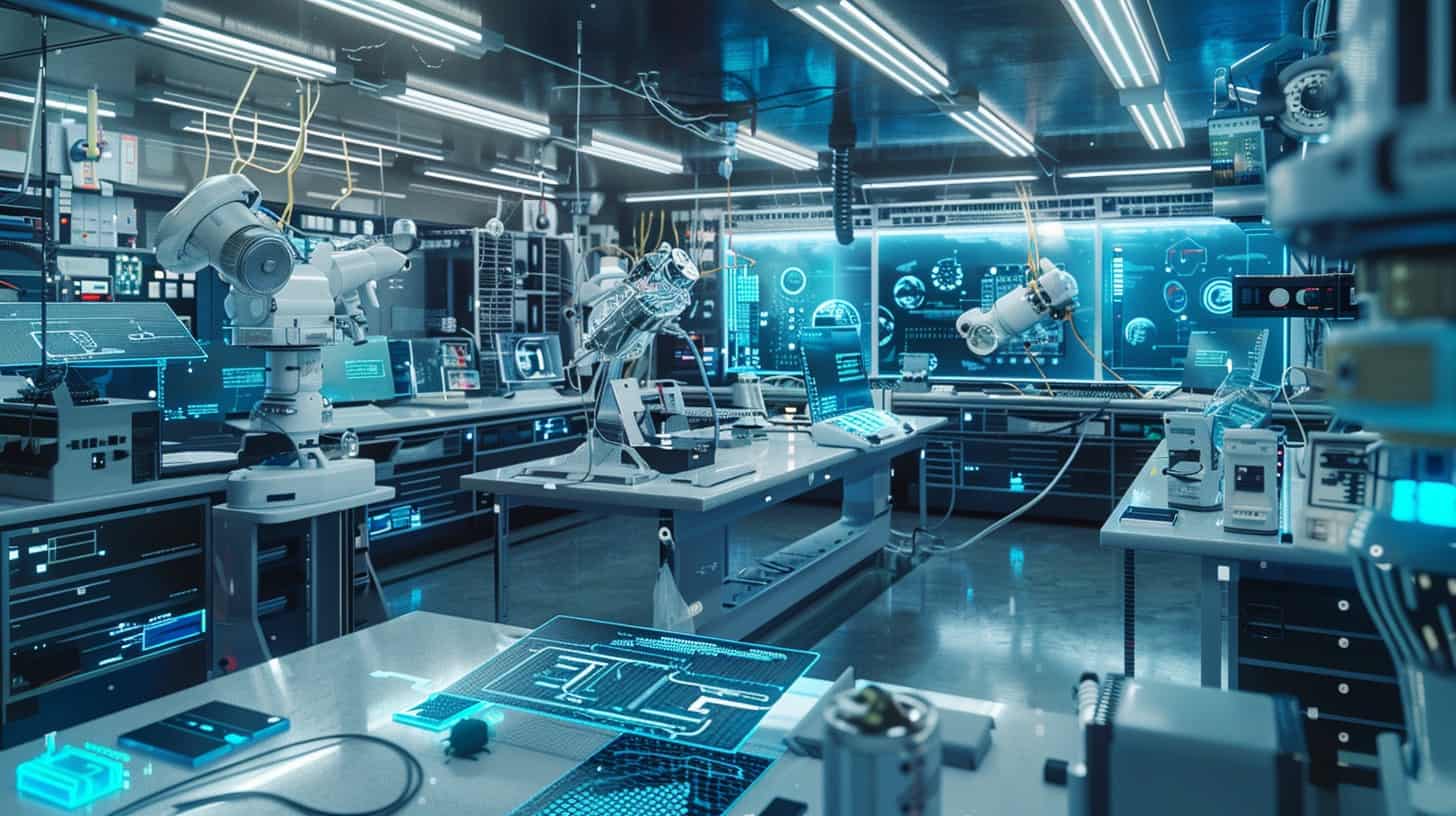
Transitioning from what computer vision entails, let’s dive into how it actually operates. At its core, computer vision strives to mimic human visual perception—this involves a lot more processing and the ability to analyze entire images or sequences of images.
Through the integration of artificial intelligence and machine learning techniques, these systems learn from a plethora of training data sets. They’re not just looking at pictures; they’re identifying patterns, making sense of various shapes, and understanding contexts, much like our brains do when we see something.
The process kicks off with digital images or videos being fed into the system from cameras or archives. Algorithms then break down these visuals into manageable pieces for analysis.
Think of this as teaching a computer to see—the algorithms use statistical principles and unsupervised learning methods to classify and segment objects within an image. Whether it’s recognizing faces in a crowded room or differentiating between vehicles on the road, every task is about decoding pixels to make sense of the world visually without any human intervention.
This advanced capability is why we now have applications ranging from self-driving cars to automated retail checkout systems – all thanks to computers gaining insight akin to human eyesight yet operating at an unparalleled scale and speed.
Applications of Computer Vision
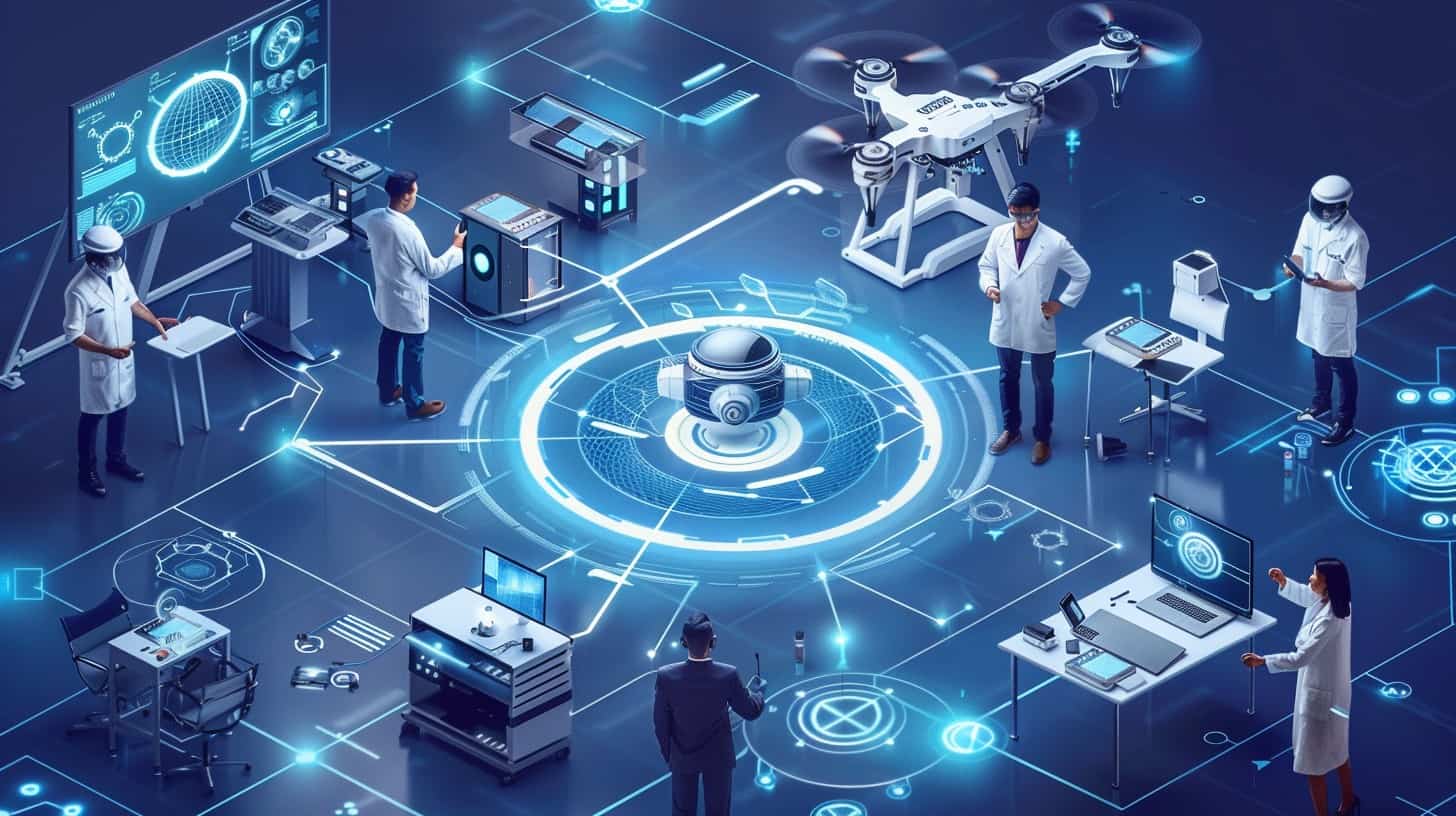
From making self-driving cars a reality to transforming how retailers manage their inventory, computer vision is shaping the future—dive in to explore more.
Augmented Reality
Augmented reality, or AR, changes how we see the world around us. It uses computer vision and machine learning to enhance our environment with digital overlays. Imagine looking through your phone and seeing directions on the ground in front of you, or playing a game where fantasy creatures pop up right in your living room.
This is what AR can do.
The combo of computer vision and machine learning has really pushed AR forward. Now, tasks like image classification, object detection, and segmentation are more accurate than ever.
This means that when I use an AR app to measure my living room for a new couch or to try on virtual makeup, it’s more precise and realistic. Augmented reality isn’t just cool tech—it’s changing how we interact with everything from retail to gaming.
Self-Driving Cars
Shifting from augmented reality to the realm of autonomy, self-driving cars stand as a testament to the fusion of computer vision and machine learning. These automated vehicles rely heavily on computer vision to identify obstacles and navigate traffic, seamlessly integrating machine learning for data analysis and response.
The synergy between these technologies has not only enhanced accuracy but also improved performance significantly.
Self-driving cars use an array of sensors and digital cameras to track and recognize objects in real time. Machine learning processes vast amounts of traffic data and road conditions, enabling these vehicles to make informed decisions on the fly.
This advanced tech allows for obstacle detection and automated navigation, marking substantial advancements in autonomous driving capabilities—fueled by deep learning applications tied closely with artificial intelligence-based systems.
Retail
Retail is changing fast, thanks to computer vision and machine learning. Stores use these technologies for smarter warehouse automation and just-in-time stock management. This means they can keep exactly what customers want in stock, without wasting space or resources.
Computer vision helps track products as they move from the back room to the sales floor. It also speeds up checkouts and improves customer service by recognizing items instantly—no more long waits at the cash register.
Big names like Alibaba are already using this tech to revolutionize shopping, making it faster and more efficient than ever before.
Warehouse Management
Moving from retail to warehouse management, the shift is towards efficiency and productivity. Computer vision plays a crucial role here. It streamlines warehouse automation and just-in-time stock operations.
This tech not only speeds things up but also cuts down on mistakes. Think robots sorting packages faster than any human could, or software keeping track of inventory in real time.
Tech giants and startups alike are jumping on this bandwagon, using computer vision and machine learning to transform how warehouses operate. These advancements allow for more accurate image classification, object detection, and segmentation—key processes in managing inventories effectively.
The result? A revolution in the industry that boosts automation, enhances accuracy, and optimizes logistics like never before.
Comparing Machine Vision and Computer Vision
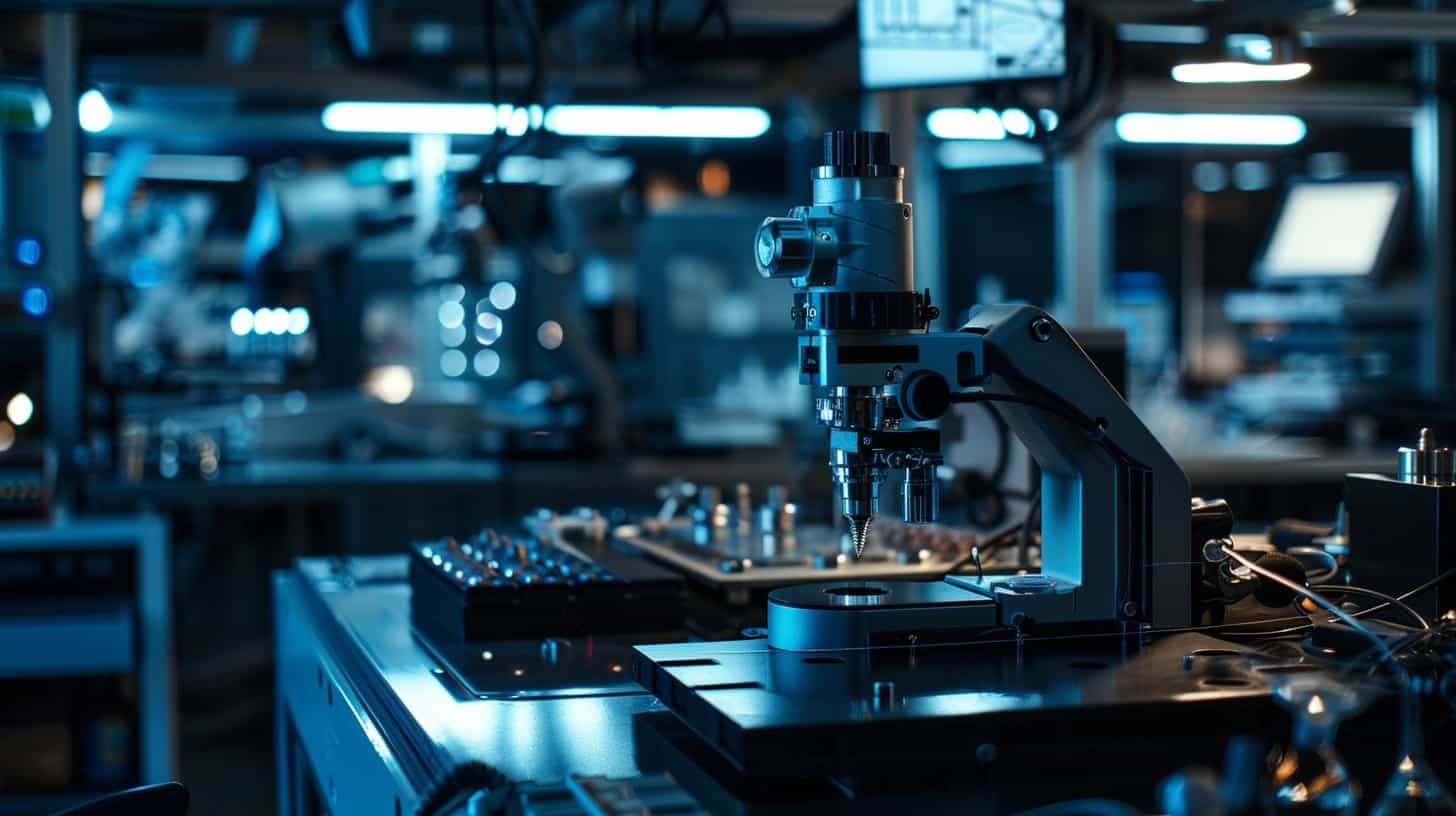
Machine vision and computer vision might sound similar, but they tackle different challenges. While one focuses on real-time processing for industrial tasks, the other dives deep into understanding images to mimic human sight.
Technology Differences
Talking geek to geek, let’s dive straight into the tech differences between machine vision (MV) and computer vision (CV). First off, MV leans heavily on specialized cameras and specific software for its magic.
It zeroes in on particular details within an image to carry out tasks like inspections in manufacturing or guiding industrial robots. Think of it as having laser focus – it needs its own gear but excels in precision.
On the flip side, CV is more of a free spirit. It doesn’t rely solely on specialty cameras. Instead, it can process and analyze visual content from various sources—digital cameras, videos, even live feeds.
Its strength lies in understanding the bigger picture from these inputs without needing custom hardware or integration. Whether we’re talking about augmented reality apps or autonomous cars navigating streets, CV works independently with a broader perspective.
Focus Differences
Machine vision zooms in on specific details within an image to perform tasks with precision. It’s all about sticking to the rules, following set parameters for automated tasks like checking barcodes in a warehouse or inspecting parts on a manufacturing line.
Here, the focus is narrow but deep – think quality control where every millimeter matters.
On the flip side, computer vision casts a wider net. It dives into entire images or even video feeds to understand and interpret what’s there. This tech powers AI-driven cars that need to make sense of their surroundings and Augmented Reality (AR) games that merge virtual objects with the real world.
Computer vision doesn’t just look; it sees and comprehends, turning visual inputs into actionable insights without confinement to predefined aspects.
Real-time vs historical images
Shifting gears from focus differences, let’s dive into how machine vision and computer vision differ in their approach to image processing. Machine vision hinges on capturing images in real-time.
This is crucial for applications like robot vision or quality control on manufacturing lines, where split-second decisions are made based on the latest visual data. The system grabs these fresh images using advanced cameras and immediately analyzes them to guide actions or make judgments.
On the flip side, computer vision thrives on historical images. It’s all about digging through past visual content to train algorithms, allowing them to recognize patterns and objects over time.
Whether it’s facial recognition software learning from thousands of faces or agricultural equipment distinguishing between crops and weeds, computer vision systems digest a wealth of stored images.
They loop through this data repeatedly until they become adept at task automation—essentially learning from history to predict or interpret the visual world around us.
FAQs About Machine Vision vs Computer Vision
What’s the difference between machine vision and computer vision?
Machine vision uses cameras and computers to complete specific tasks, like checking products on an assembly line. Computer vision is broader, letting computers understand images and videos, much like how humans see.
Can both be used in tech jobs?
Yes! From game developers creating more realistic environments to engineers improving self-driving cars—both fields offer lucrative tech jobs in artificial intelligence (AI), robotics, and digital systems.
How do they apply to everyday items?
Well, consider your smartphone – it uses computer vision for facial recognition; while machine vision might be used in making that phone, ensuring each part is perfectly placed during assembly.
Do they only work with images?
Not at all! Beyond images, these technologies handle object segmentation (dividing a scene into parts), speech recognition in consumer electronics, and even analyze data for digital marketing strategies.
Can either help with privacy issues?
Certainly—both play roles here. For example, computer visions can secure personal data by identifying who should access information; machine visions keep track of inventory without exposing sensitive details to human workers.
Where else might you find them outside of tech industries?
You’d be surprised—they’re everywhere! In agriculture, drones use them for crop monitoring; fintech applications rely on them for security… Even medical diagnostics benefit from their ability to process automation swiftly and accurately.